- Article
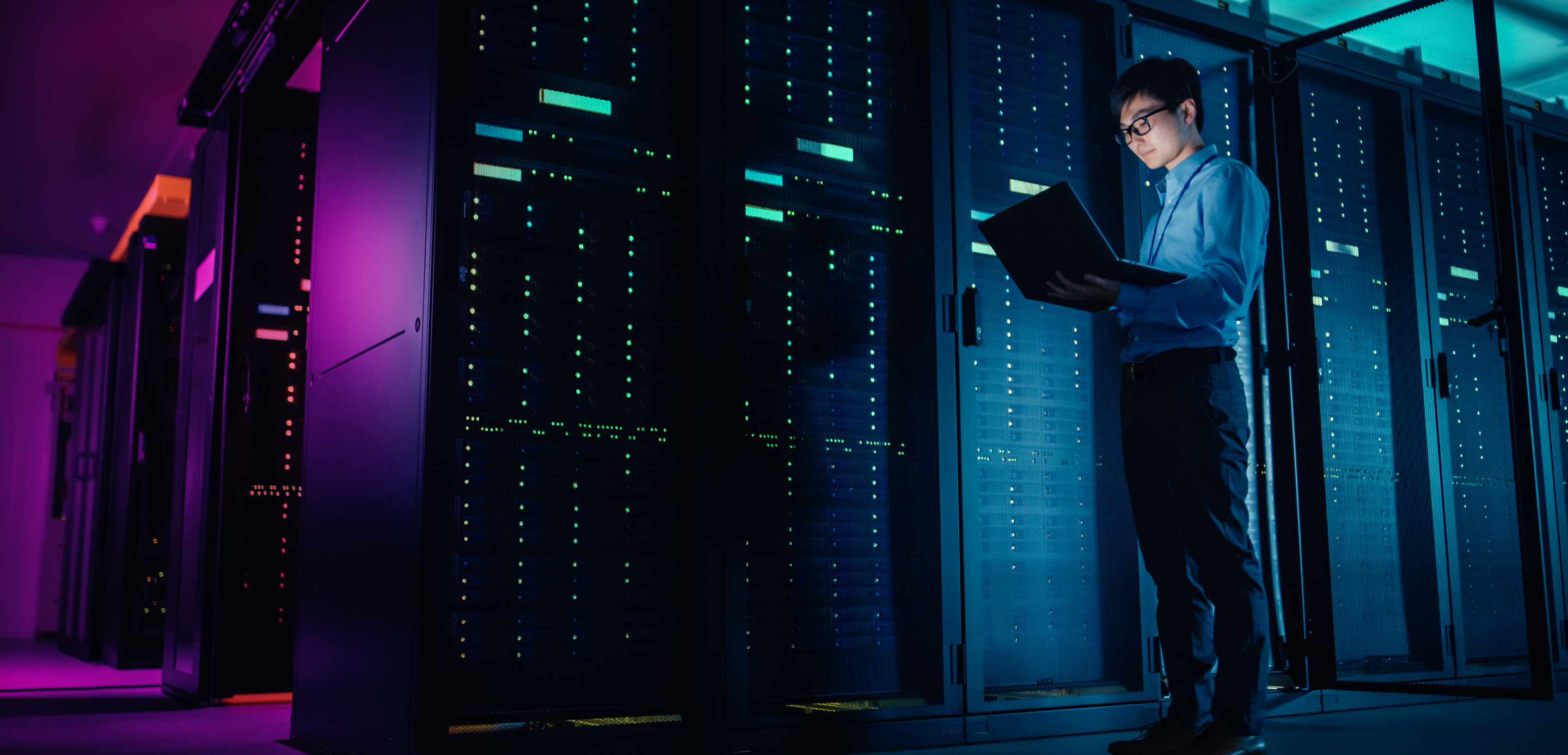
- Innovation
- Artificial Intelligence
Explaining explainable AI
When AI is seen as a ‘black box’ making opaque decisions, it’s difficult for users to trust those decisions. Explainable AI aims to change that.
Whether we realise it or not, we now interact with AI every day in a multitude of settings. It’s powering the predictions of the next word in our messaging app, it’s using image recognition to tag photos on our social media, it’s recognising our voices and what we’re saying so we can use virtual personal assistants like Siri or Alexa. Along with these everyday interactions, AI is also being used in healthcare settings to help diagnose disease, in criminal justice settings to aid police or predict the chances of reoffending, and in your bank’s decision to grant or deny a loan.
Why we need an explanation
Many of today’s decision-making tools are powered by or augmented with AI and can produce highly accurate results, but they can also be highly complex. This level of complexity can lead to a ‘black box’ situation, where it may not be intuitive to deduce what factors lead to a particular decision – even to expert users. Often this is unacceptable as users need to be able to understand and trust the system. Users should have access to enough information to know that the system:
- Is monitored regularly and working as intended
- Is safeguarded against built-in biases
- Meets regulatory standards and legal requirements
- Is assessed for vulnerabilities that may be exploited by bad actors
- Offers individuals agency in the decision-making process – such as the right to appeal a decision
Different explanations for different users
It’s clear that different users will have different needs when it comes to explainability and interpretability. An individual applying for a loan, for example, may want to appeal the decision. The developer designing that AI algorithm may want to assess multiple decisions for any hint of bias, understanding what’s driving decision-making and the true predictive performance of their algorithms. The bank will want to ensure that it can be confident it’s not offering a loan to someone who can’t pay, or refusing someone else who may take their custom elsewhere.
User types can include:
- AI Developers – e.g. those who architect, train, test, and deploy AI solutions. They need to increase their understanding of the drivers within the data that powers the tool in order to maximise its performance and fairness.
- Decision-Makers – e.g. Bank employees who are the recipients of the output of AI systems, which they use to help them complete tasks and make decisions.
- Impacted Users – e.g. those who may not directly interact with AI tools but who are ultimately affected by them, but rarely have either AI or domain expertise. In this example, it would be the loan applicant.
- Independent Review – e.g. have a particular interest in fairness, accountability and transparency of AI systems. A diverse range of AI and domain experts who are charged with reviewing and understanding the potential risks associated with AI/ML models. In this case, the bank's compliance and legal teams, as well as ombudsmen, consumer rights groups or financial regulators would all be examples.
Clearly, each group will expect different levels of detail in their explanations of AI systems. Developers will want highly technical and numerical insight into performance, confidence, and fairness, while non-experts will need to be able to understand an explanation and its real-world implications. Explainable AI needs to be able to offer:
- Outcome-based explanations – where the reasoning behind the outcome of a decision is made clear.
- Process-based explanations – where the steps taken across the design and implementation of an AI system, including the data used, its preparation, and how the system is architected to maximise the accuracy, reliability, security, fairness and robustness of its decisions and behaviours are made clear.
Within each of those forms, are further important sub-types of explanation that are relevant to different users, as shown here:
Outcome-based | Process-based |
---|---|
Rationale explanation Exploring the reasons that led to a decision, delivered in an accessible and non-technical way. Impact explanation Making explicit the steps taken across the design and implementation of the AI system to consider and monitor the impacts that the use of an AI system and its decisions has or may have on an individual, and on wider society.
| Responsibility explanation Who is involved in the development, management and implementation of an AI system, and who to contact for a human review of a decision. Data explanation What data has been used in a particular decision and how. Fairness explanation Steps taken across the design and implementation of an AI system to ensure that the decisions it supports are generally unbiased and fair, and whether or not an individual has been treated equitably. Performance explanation Steps taken across the design and implementation of an AI system to maximise the accuracy, reliability, security and robustness of its decisions and behaviours. |
Categorisation of explanation types into outcome and process based.
Are explanations enough?
We can see that different users and different contexts will create different explainability needs. Some AI systems don’t really raise concerns about explainability, such as the predictive text on your smartphone, because there’s no significant consequences from getting it wrong.
In other cases, a simple explanation about how an AI system works may not be enough to give users confidence or support accountability. Human decision-making can be similarly opaque, but that’s why there are complex systems of checks and balances, audits and second opinions, to safeguard us from human errors. Explainable AI is just one part of creating the transparency and interpretability that will allow for the same accountability when an algorithm is making the decision.
Five core design principles for explainable AI
Core principles for design can help create AI systems that are interpretable and user-friendly.
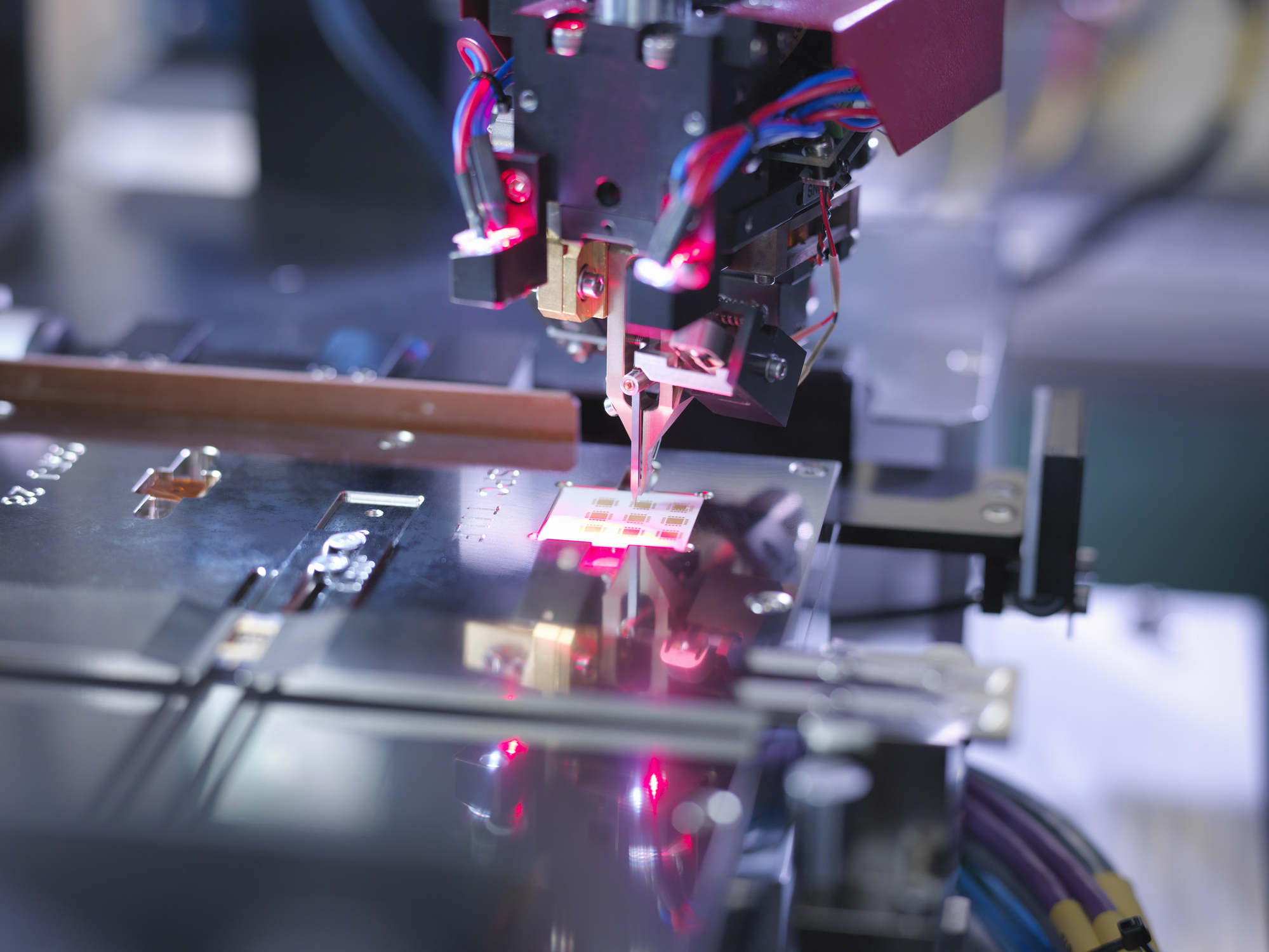